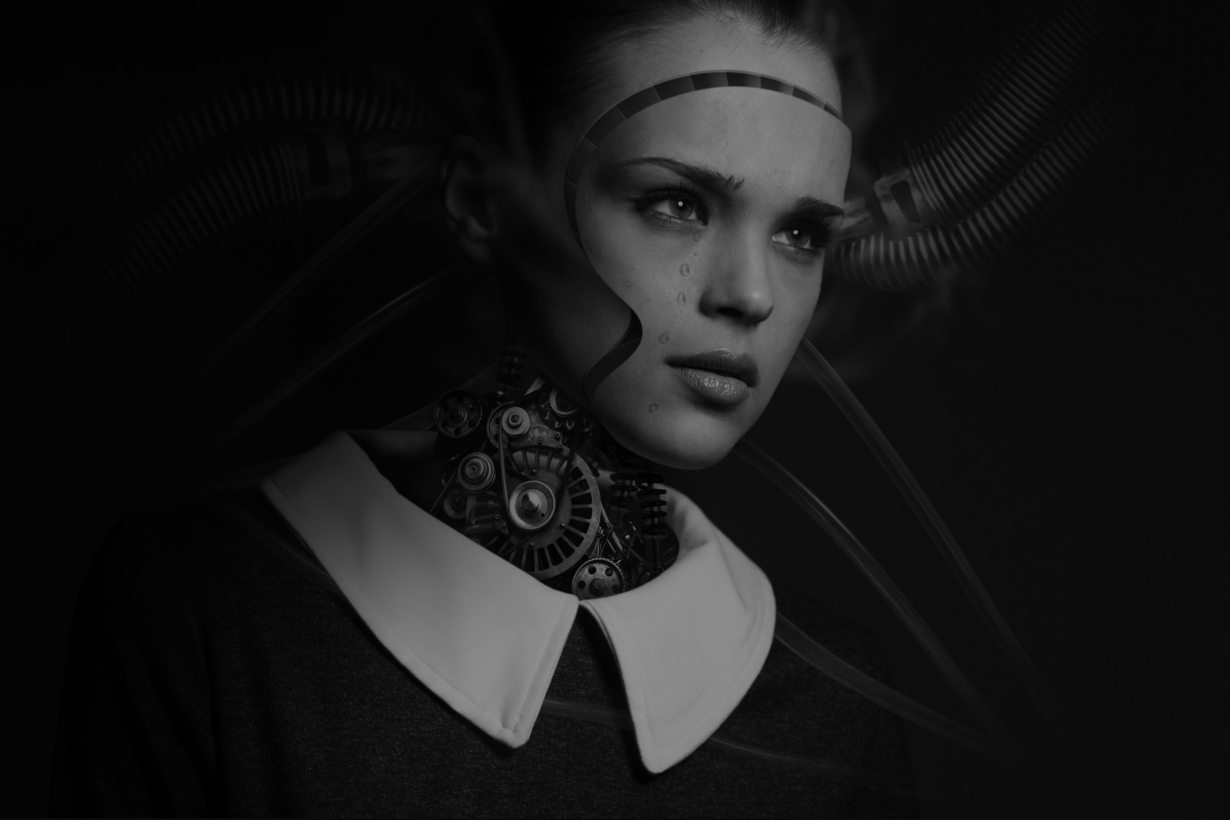
Mahrad Pisheh Var - Professional Portfolio
Leading the Future of AI and Machine Learning
Dedicated and highly motivated computer science professional with three degrees, including a Ph.D. in Computer Science, seeking opportunities to leverage my expertise in Python, Java, and C++ as well as my extensive experience in game development and machine learning.
Academic and Research Experience
Ph.D. In Computer Science at the University of Essex
2020 - 2024
Thesis: “Minimalistic Adaptive Dynamic-Programming Agents for Memory-Driven Exploration”.
Bachelor of Science in Computer Science at the University of Essex
28/06/2018
With First Class Honors, Final Project: TILE-BASED RPG from Scratch (Engine and Design) in C++.
Master’s in Computer Games at the University of Essex
22/11/2019
With Distinction, Thesis: Investigating Immersion of Environments affecting the performance of BCI.
Publications
-
This paper describes a simple memory augmentation technique that employs tabular Q-learning to solve binary cell structured mazes with exits generated randomly at the start of each solution attempt. A standard tabular Q-learning can solve any maze with continuous learning; however, if the learning is stopped and the policy is frozen, the agent will not adapt to solve newly generated exits. To avoid using Recurrent Neural Networks RNNs to solve memory-required tasks, we designed and implemented a simple external memory to remember the agent’s cell visit history. This memory also expands the state information to hold more information, assisting tabular Q-learning in distinguishing its path from entering and exiting a maze corridor. Experiments on five maze problems of varying complexity are presented. The maze has two and four predefined exits; the exit will be randomly assigned at the start of each solution attempt. The results show that tabular Q-learning with a frozen policy can outperform standard deep-learning algorithms without incorporating RNNs into the model structure. https://link.springer.com/chapter/10.1007/978-3-031-37717-4_22
-
This article presents a scenario where a simple simulated organism must explore and exploit an environment containing a food pile. The organism learns to make observations of the environment, use memory to record those observations, and thus plan and navigate to the regions with the strongest food density. We compare different reinforcement learning algorithms with an adaptive dynamic programming algorithm and conclude that backpropagation through time can convincingly solve this recurrent neural-network challenge. Furthermore, we argue that this algorithm successfully mimics a minimal ‘functionally sentient’ organism’s fundamental objectives and mental environmental-mapping skills while seeking a food pile distributed statically or randomly in an environment. https://journals.sagepub.com/doi/full/10.1177/10597123231166416
-
Adaptive Dynamic Programming (ADP) and Reinforcement Learning (RL) are pivotal frameworks in machine learning, each presenting unique benefits and hurdles. This thesis examines the performance and adaptability of ADP agents using Backpropagation Through Time (BPTT) in continuous spaces. A Memory-Based Backpropagation Through Time (MBPTT) is reviewed, enhancing the conventional BPTT approach by integrating memory mechanisms to refine decision-making in partially observable environments. Drawing upon foundational and recent developments in RL and ADP, this study explores the capability of BPTT agents across various environmental settings. It critically assesses different algorithms and memory models, including Long Short-Term Memory (LSTM) and Gated Recurrent Units (GRU), in simulating a ``functionally sentient'' organism seeking food. The research makes two main contributions. Firstly, it empirically shows that even the simplest forms of memory-augmented agents can effectively navigate through a maze, performing better than existing techniques. This highlights the practical use of memory-based algorithms in spatial tasks. Secondly, the study investigates the performance of Backpropagation Through Time (BPTT) in bicycle navigation. It introduces a simulated organism that successfully combines BPTT with memory functions, demonstrating efficiency in environmental mapping and food search tasks. This work provides a solid foundation for future research in integrated learning systems. In conclusion, this thesis reconciles the theoretical distinctions between memory and adaptive dynamic programming. Combining theoretical understanding with practical applications contributes to the ongoing effort to create more resilient, efficient, and adaptive agents in the rapidly advancing field of machine learning. https://repository.essex.ac.uk/38575/